Healthcare AI: The Power Of Data Annotation
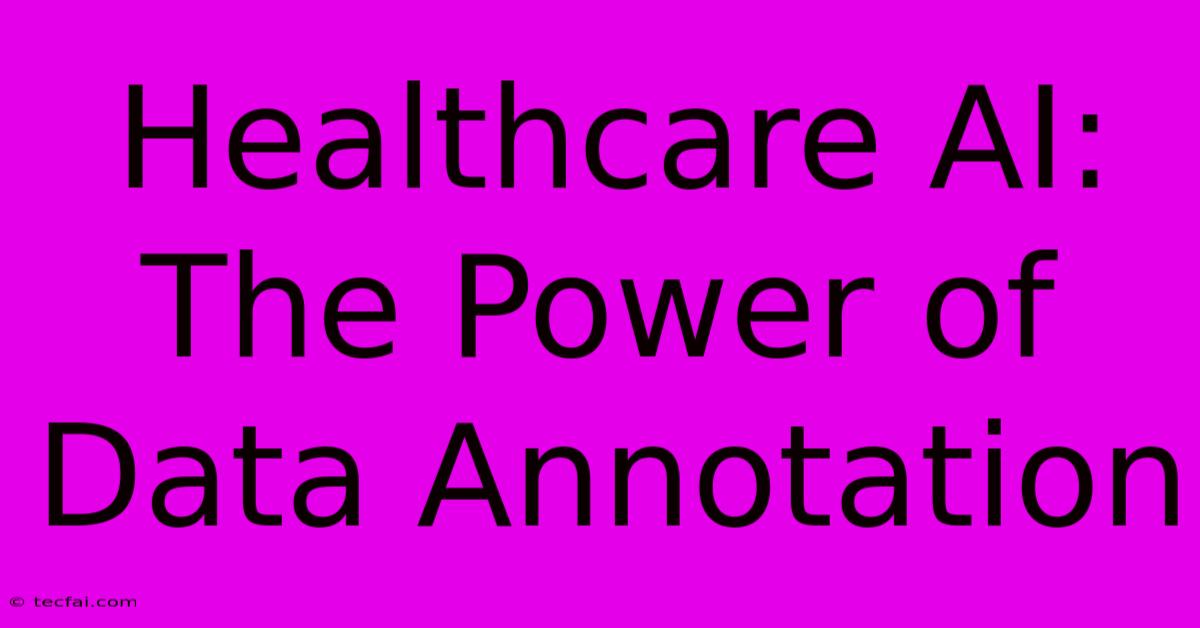
Discover more detailed and exciting information on our website. Click the link below to start your adventure: Visit Best Website tecfai.com. Don't miss out!
Table of Contents
Healthcare AI: The Power of Data Annotation
Artificial intelligence (AI) is rapidly transforming healthcare, offering the potential to improve diagnostics, personalize treatment, and streamline operations. However, the power of AI in healthcare hinges on one crucial element: high-quality data. And that's where data annotation comes in. This process, often overlooked, is the unsung hero behind the success of AI in medicine. This article explores the critical role of data annotation in powering healthcare AI, discussing its various types, challenges, and future implications.
What is Data Annotation in Healthcare?
Data annotation is the process of labeling and tagging raw data to make it understandable and usable for AI algorithms. In healthcare, this raw data can take many forms, including:
- Medical images: X-rays, CT scans, MRIs, and pathology slides require annotation to identify anatomical structures, lesions, and other relevant features.
- Electronic health records (EHRs): EHRs contain a wealth of information, but it needs to be tagged to extract meaningful insights related to diagnoses, treatments, and patient outcomes.
- Clinical notes: Free-text clinical notes require annotation to identify key medical entities like medications, diagnoses, and procedures.
- Biosignals: Data from wearable sensors and other devices needs annotation to correlate physiological signals with health conditions.
- Genomic data: Analyzing genomic sequences requires annotation to identify genes, mutations, and variations.
Each type of data requires specific annotation techniques. For example, medical images might use bounding boxes to highlight regions of interest, while text annotation might involve named entity recognition (NER) to identify and classify medical terms.
Types of Data Annotation in Healthcare
Several annotation techniques are crucial for developing robust AI models in healthcare:
- Image Annotation: This includes bounding boxes, polygons, semantic segmentation, and landmark annotation for precise identification of features within medical images.
- Text Annotation: This involves NER, relationship extraction, sentiment analysis, and topic classification to extract meaningful information from clinical notes and other textual data.
- Audio Annotation: This is used for transcribing and classifying audio data, such as patient consultations or heart sounds, aiding in diagnosis and monitoring.
- Video Annotation: This involves tracking and labeling objects and events within medical videos, such as surgical procedures or patient movement analysis.
Why is Data Annotation Crucial for Healthcare AI?
High-quality data annotation is paramount for several reasons:
- Improved Accuracy: Precisely annotated data trains AI algorithms to make more accurate diagnoses and predictions.
- Enhanced Model Performance: Well-annotated data leads to better-performing AI models, ultimately improving patient care.
- Faster Development Cycles: Efficient annotation processes streamline the development and deployment of AI-powered healthcare solutions.
- Reduced Errors: Thorough annotation minimizes errors in AI model training, leading to more reliable results.
- Data Security and Privacy: Proper annotation practices ensure compliance with data privacy regulations like HIPAA.
Challenges in Healthcare Data Annotation
Despite its importance, healthcare data annotation presents unique challenges:
- Complexity of Medical Data: Medical data is often complex, requiring specialized knowledge and expertise for accurate annotation.
- Data Variability: Data variability across different sources and institutions can complicate the annotation process.
- Data Scarcity: The availability of large, high-quality annotated datasets can be a significant bottleneck.
- Cost and Time: Data annotation is a labor-intensive and time-consuming process, which can be expensive.
- Maintaining Annotation Consistency: Ensuring consistent annotation across different annotators is critical to prevent bias and improve accuracy.
The Future of Data Annotation in Healthcare
The future of data annotation in healthcare is likely to involve:
- Increased Automation: The use of automated annotation tools and techniques will help address the challenges of cost and time.
- Active Learning: Active learning techniques will focus annotation efforts on the most informative data points, improving efficiency.
- Synthetic Data Generation: Generating synthetic data can augment real-world datasets, addressing data scarcity issues.
- Federated Learning: Federated learning allows training AI models on decentralized data sources, preserving data privacy while improving model performance.
In conclusion, data annotation is the cornerstone of successful healthcare AI. Addressing the challenges and embracing innovative techniques will unlock the full potential of AI to improve patient outcomes and revolutionize healthcare delivery. As AI continues to mature within the healthcare sector, the importance of meticulously annotated data will only continue to grow.
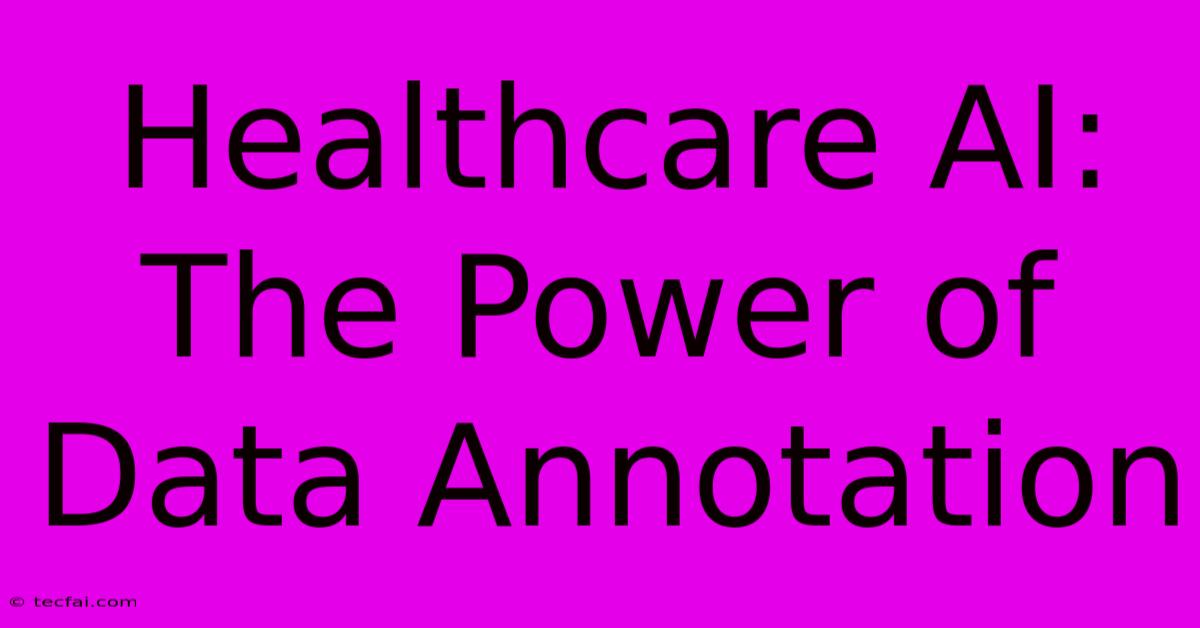
Thank you for visiting our website wich cover about Healthcare AI: The Power Of Data Annotation. We hope the information provided has been useful to you. Feel free to contact us if you have any questions or need further assistance. See you next time and dont miss to bookmark.
Featured Posts
-
Bob Bryar My Chemical Romance Dies Aged 44
Nov 30, 2024
-
Navigate Supply Chain Disruptions Essential Skills
Nov 30, 2024
-
Auckland Fc Extends Winning Streak Against Newcastle
Nov 30, 2024
-
High Turnout In County Roscommon
Nov 30, 2024
-
Turning Data Into Insights
Nov 30, 2024