AI Healthcare: Data Annotation's Impact
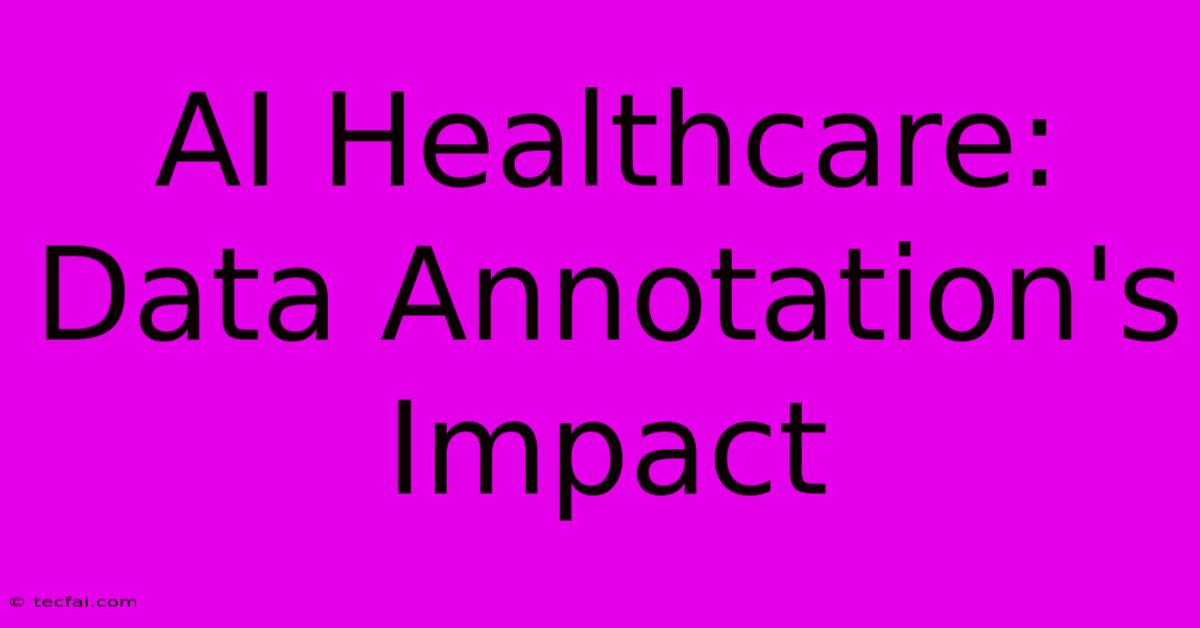
Discover more detailed and exciting information on our website. Click the link below to start your adventure: Visit Best Website tecfai.com. Don't miss out!
Table of Contents
AI Healthcare: Data Annotation's Impact
The healthcare industry is undergoing a dramatic transformation, driven by the rapid advancements in Artificial Intelligence (AI). From diagnosing diseases to personalizing treatment plans, AI is poised to revolutionize how we approach healthcare. However, the success of AI in healthcare hinges critically on one often-overlooked component: data annotation. This crucial process forms the foundation upon which accurate and effective AI algorithms are built.
Understanding Data Annotation in AI Healthcare
Data annotation is the process of labeling and tagging data to make it understandable to AI algorithms. In the context of healthcare, this data can include medical images (X-rays, CT scans, MRIs), electronic health records (EHRs), genomic data, and wearable sensor readings. Without accurate and comprehensive annotation, AI models will fail to learn effectively, leading to inaccurate diagnoses, ineffective treatments, and potentially harmful outcomes.
For example, annotating a chest X-ray might involve identifying and labeling areas indicating pneumonia, lung cancer, or other abnormalities. Similarly, annotating EHR data could involve labeling specific patient demographics, diagnoses, medications, and treatment responses. The accuracy and consistency of this annotation directly impact the AI model's ability to accurately identify patterns and make informed predictions.
The Types of Data Annotation Used in AI Healthcare
Several types of data annotation are employed in AI healthcare, each tailored to the specific type of data and AI application:
-
Image Annotation: This involves labeling objects, regions, and boundaries within medical images. Common techniques include bounding boxes, polygons, semantic segmentation, and landmark annotation. This is crucial for applications like image-based diagnostics and surgical planning.
-
Text Annotation: This focuses on labeling textual data within EHRs and medical reports. Techniques include named entity recognition (NER), relationship extraction, and sentiment analysis. This is vital for tasks like automated report generation and patient risk assessment.
-
Audio Annotation: This involves labeling audio data, such as heart sounds or respiratory patterns. This is crucial for developing AI-powered diagnostic tools that can analyze audio signals for detecting abnormalities.
-
Video Annotation: This is used to label actions and events in videos, such as surgical procedures. This helps train AI models for surgical assistance and robotic surgery applications.
The Impact of High-Quality Data Annotation
The impact of high-quality data annotation on AI healthcare cannot be overstated. It directly influences:
-
Accuracy of AI models: Accurate annotation leads to AI models that provide more precise diagnoses and predictions.
-
Improved efficiency: Automated analysis of annotated data can significantly speed up diagnostic processes and reduce the workload on healthcare professionals.
-
Enhanced patient care: By enabling earlier and more accurate diagnoses, AI can lead to improved patient outcomes and reduced mortality rates.
-
Drug discovery and development: AI can accelerate drug discovery by analyzing large datasets of annotated genomic and molecular data.
-
Personalized medicine: AI models trained on annotated data can enable the development of personalized treatment plans tailored to individual patient needs.
Challenges in Data Annotation for Healthcare
Despite its importance, data annotation in healthcare faces several challenges:
-
Data privacy and security: Healthcare data is highly sensitive, requiring robust security measures to protect patient privacy.
-
Data variability and complexity: Medical data can be highly variable and complex, making accurate annotation a challenging task.
-
Cost and time: Annotating large datasets can be expensive and time-consuming, requiring specialized expertise.
-
Inter-annotator agreement: Ensuring consistency among different annotators is crucial to maintain data quality.
The Future of Data Annotation in AI Healthcare
The future of AI in healthcare is inextricably linked to the advancements in data annotation. We can expect to see:
-
Increased automation: The development of automated annotation tools will help reduce costs and improve efficiency.
-
Improved annotation techniques: New techniques and algorithms will be developed to improve the accuracy and speed of annotation.
-
Focus on data quality: Greater emphasis will be placed on ensuring the quality and consistency of annotated data.
In conclusion, data annotation is not merely a supporting process but a cornerstone of successful AI implementation in healthcare. By addressing the challenges and embracing advancements in this field, we can unlock the full potential of AI to improve patient care and revolutionize the healthcare industry. The future of AI healthcare is bright, but it depends on the continued development and refinement of accurate and reliable data annotation techniques.
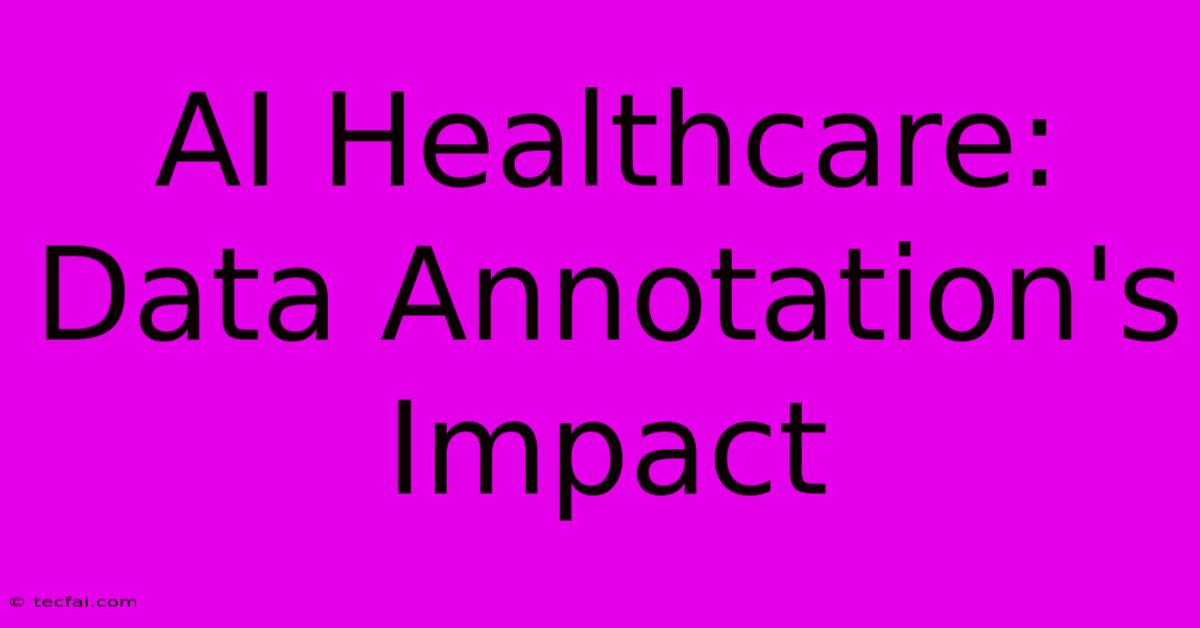
Thank you for visiting our website wich cover about AI Healthcare: Data Annotation's Impact. We hope the information provided has been useful to you. Feel free to contact us if you have any questions or need further assistance. See you next time and dont miss to bookmark.
Featured Posts
-
Movie Review The Agency Fails
Nov 30, 2024
-
Match Preview Sheffield Utd Sunderland
Nov 30, 2024
-
50 Off Sephora Bestsellers Black Friday
Nov 30, 2024
-
Assemblyman Ng Penang Hindi Totoo Ang Sinabi Ko Kay Anwar
Nov 30, 2024
-
Herdmans Tfc Departure Footy Prime
Nov 30, 2024